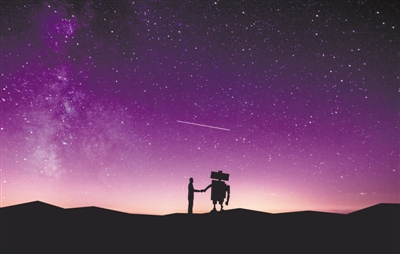
Looking for dark matter, machines are better than human eyes. A recent paper published in Computational Astrophysics and Cosmology shows that the deep learning AI framework jointly developed by Lawrence Berkeley National Laboratory (hereinafter referred to as Berkeley Laboratory) can explore the signs of dark matter in the universe.
In recent years, artificial intelligence has been increasingly applied to astronomical research. Deep learning requires massive amounts of data, and astronomy is the field where AI shows its talents. Machines can find a needle for human beings from the vast ocean and capture new stars, new extraterrestrial planets and even dark matter.
Recognizing the "gravitational lens", AI has made contributions.
Finding "gravitational lens" is the basic method to study the distribution of dark matter. An object with a huge mass will distort the passing light like a lens, and if we find out this distortion, we can capture the non-luminous mass.
The paper shows that CosmoGAN, a deep learning AI framework established by Berkeley Lab, can analyze the relationship between gravitational lens and dark matter. It can create high fidelity and weak gravitational lens convergence diagram.
Once upon a time, it was troublesome to find the simulation and data processing needed for the "gravitational lens". Twenty scientists spent months looking at only a small space image. Physical simulation takes billions of computing hours and takes up megabytes of disk space.
The progress of neural network provides opportunities. The team led by Berkeley Lab introduced a "generative confrontation network (GANs)". Researcher Mustafa said: "There are other deep learning methods that can get convergence graphs from many images, but compared with competitive methods, GANs generates very high-resolution images while still having the high efficiency of neural networks."
Now, astronomers can use CosmoGAN to analyze a much larger sky area, and the speed is faster.
CosmoGAN is not the only astronomical deep learning neural network that has made progress. For example, the University of Toronto used deep learning technology to analyze satellite images of lunar craters, and the neural network of P8 supercomputer discovered 6,000 new craters in just a few hours, twice the number of craters discovered by humans in the past decades. The University of Illinois at Urbana-Champaign uses deep learning to detect and analyze gravitational waves of black hole collisions. AI blooms everywhere in astronomy.
There is too much data to play without a machine.
In the past few years, most directions in the field of astronomy have tried to use artificial intelligence. Considering the amount of data that astronomy has to deal with, this is a natural idea. Let the machine practice to analyze clues, otherwise, astronomy in the future will not work.
The 2019 GPU Technology Conference held not long ago attracted artificial intelligence scholars all over the world. Brent Robertson, an astronomer from the University of California, Santa Cruz, was invited to give a speech at the conference. He pointed out: "Astronomy is at the cusp of a new data revolution". Robertson believes that the new generation of astronomical instruments must cooperate with the new generation of software driven by deep learning.
For example, the large-caliber all-day patrol telescope (LSST) is expected to run in three years. It toured 37 billion galaxies in the southern half of the universe, generating a ten-year uninterrupted video. LSST is equipped with a 3.2 billion pixel camera, which generates 25TB of data every night, which is equivalent to all the data contributed by the advanced astronomical telescope in a lifetime.
Another example is the Square Kilometer Array Radio Telescope (SKA). It is spread all over the world, some antennas are deployed in eight countries in southern Africa, and more than one million antennas are located in Australia and New Zealand. Its raw data reaches 5000 PB every day, and there are about 50 PB after processing.
"Dark Energy Survey" compiles star maps of hundreds of millions of galaxies; "Gaia" satellite surveying and mapping stars with galactic coefficient of one billion; The "Zwicky" project can scan 3,750 square degrees of sky every hour. In China, the daily data volume of FAST will reach 150TB;; Guo Shoujing telescope has observed 9.01 million spectra, which is the largest celestial spectrum library in the world … …
Capture patterns that humans can’t see.
There are more and more data, and scientists try to aggregate them. However, at the GPU conference, Robertson said that in the future, several large astronomical telescopes will produce a large amount of data together, which is too complicated for human beings to directly use after aggregation. Scientists at the University of California, Santa Cruz, tried to solve this problem. Morpheus deep learning framework created by a doctoral student in computer science department can classify celestial bodies pixel by pixel based on the original data of telescope.
Scientists at the University of California, Santa Cruz, also use AI to better study the formation of galaxies. In a study published in early 2019, scientists trained computers with computer-simulated galaxies to learn three key stages of galaxy evolution. Later, computer analysis of galaxy images from the Hubble Space Telescope performed surprisingly well.
Artificial intelligence is applied to face recognition. After training with massive data, you can recognize this person’s makeup and old age according to a photo. And many images in the universe can be classified in the same way.
"Deep learning can look for patterns, and machines can see very complicated patterns that humans can’t see." David Ku, a scientist involved in the research, said, "We hope to further test this method. In the proof-of-concept study, the machine seems to have successfully found the different stages of galaxy evolution determined in the simulation in the data. "
Help astronomers find another solar system
A report at the end of 2018 showed that Google artificial intelligence made efforts to find new planets from Kepler’s exoplanet observation database. Planets are hard to find. Kepler satellite in space observes 145,000 sun-like stars, and finds planets from the faint changes of star brightness. The data recorded for four years includes about 35,000 suspected planetary records. Astronomers use machines combined with human eyes to identify, but the darkest and weakest signals are often ignored.
With the help of Google AI, we discovered two new planets, Kepler 90i and Kepler 80g. Kepler 90 was also recognized as the first exogalaxy with at least eight planets.
Neural network and machine learning processed 14 billion data points, and then successfully screened out candidates.
NASA and Google say that new technologies will find more exoplanets in the future. NASA also said that there is no need to worry about astronomers losing their jobs. Jesse Dotson, a scientist at NASA, explained that astronomers need to classify data before they are provided to neural networks, so that artificial intelligence can learn and analyze new information from them.
Dotson said: "AI will definitely work with astronomers in the future and become an indispensable tool."
Of course, machine learning also brings the risk of "black box": we get the answer, but we don’t know why the machine makes such a judgment, and maybe the answer is wrong. Machines make mistakes. Astronomers will continue to train and adapt to it.
Extended reading
Expert comments
Deep learning does not have "physical intuition"
Indeed, artificial intelligence has now penetrated into all branches of astronomy and astrophysics. At present, Lawrence Berkeley National Laboratory in the United States can quickly judge the basic cosmological constants such as dark matter and dark energy according to the three-dimensional density distribution of the universe by using deep learning. They found that after applying artificial intelligence, the statistical error is much smaller than that of the traditional statistical methods. In addition, we also use deep learning to search for hydrogen and carbon elements in the early universe in the spectrum with extremely low signal-to-noise ratio, and find that it is better than the traditional method.
At the same time, astronomers are also applying deep learning to help us judge the three-dimensional position and distance of celestial bodies, and then outline the large-scale structure of three-dimensional space. It is found that deep learning may be stronger than the traditional methods we used before in mining data and information. Artificial intelligence has also been applied to the field of detecting exoplanets by Google, and several exoplanets … … It can be said that artificial intelligence is now widely used in the frontier field of astrophysics.
But from the physicist’s point of view, artificial intelligence based on deep learning may also have its limitations. This limitation is that it can only play a role in a specific field that has been very clearly defined based on data. Only under the guidance of physicists, we can make the error bar of statistics smaller and estimate a certain quantity more accurately, but at present we can’t guide us to discover the new physical laws behind the data. Nor does it have a "physical intuition" based on beauty, symmetry and simplicity that is unique to humans.
To give a simple example, for example, Kepler’s observation data based on Tycho can find Kepler’s third law, and even the best machine learning and artificial intelligence algorithms at present may be difficult to repeat this discovery based on the same data.
Therefore, I think that the essence of the application of deep learning in astronomy is still limited to doing better statistics and fitting.
(Professor Cai Wei, Department of Astronomy, Tsinghua University)